Navigation:
Biomechatronics
- Legs
- Body
- Muscles
- Sensors
- Different configurations
Adaptive neural locomotion control for insect-like, energy-efficient, and adaptable locomotion
Self-adaptive reservoir for delay temporal memory tasks
Virtual muscles for muscle-like behavior
Reactive backbone joint control for climbing behavior
Multiple chaotic central pattern generators for locomotion generation and leg damage compensation
Performance of AMOS II
Description of AMOS II
- Mechanics
- Electronics
- Programming
Photos of AMOS II
Publications
News of AMOS II
|
Descriptions:
Open-source multi sensori-motor
robotic platform AMOS II
|
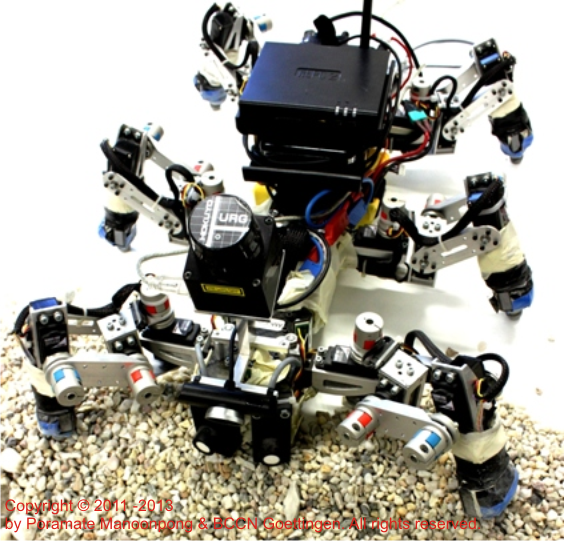 |
|
Copyright © 2011 -2013 by Poramate Manoonpong & BCCN Goettingen. All rights reserved.
The AMOS II (Advanced Mobility Sensor Driven-Walking Device version II) is a new version of AMOS. AMOS II is a biologically inspired hardware platform. It was developed in collaboration with Fraunhofer Institute for Intelligent Analysis and Information Systems IAIS
under Emmy Noether research project. It is used to study the coordination of many degrees of freedom, to perform experiments with neural control, memory, and learning and to develop artificial perceptionaction systems employing embodied control techniques. The motors allow AMOSII to carry a
maximum payload of 6 kg while walking. The size of AMOSII is 30 cm wide, 40 cm long, 20 cm high. AMOSII has a number of proprioceptive and
exteroceptive sensors, e.g., angle sensors, force sensors, current sensors, light sensors, infrared sensors, ultrasonic sensors. These sensors allow
AMOSII to autonomously perform a broad behavioral repertoire including foothold searching, elevator
reflex (swinging a leg over obstacles), self-protective reflex (standing in an upside-down position),
obstacle avoidance, escape responses, phototaxis (turn towards a light
source), climbing over obstacles, and five different gaits. Furthermore it can learn to adapt its walking pattern to a new situation. This robot can serve as a hardware platform for experiments concerning the function of a neural perception-action system. Its neural controller can be applied to advanced prosthetic and orthotic devices to generate more adaptive walking and motor planning.
![]() |
Biomechatronics
Legs
Each leg has three joints (three DOF): the thoraco-coxal (TC-) joint enables forward (+) and backward (-) movements, the coxa-trochanteral (CTr-) joint enables elevation (+) and depression (-) of the leg, and the femur-tibia (FTi-) joint enables extension (+) and flexion (-) of the tibia The morphology of this multi-jointed leg is modeled on the basis of a cockroach leg but the tarsus segments are ignored. Each tibia part contains a spring damped compliant element to absorb an impact force as well as to measure the ground contact event during the walk. In addition, passive couplings are installed
at each joint in order to yield passive compliances and to
protect a motor shaft. This high mobility of the legs enables the walking machine to walk in omnidirection and to perform a reflex behavior, e.g., standing in an upside-down position [5].
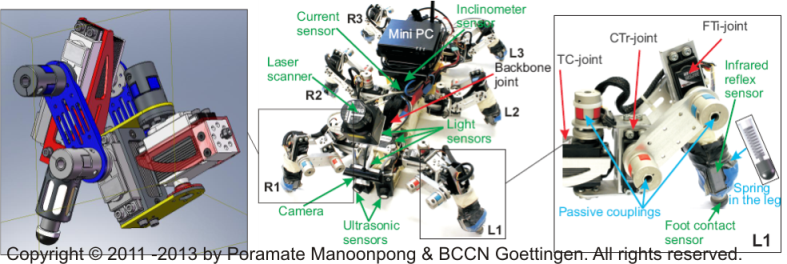
Body
Inspired by invertebrate morphology of the American cockroach’s trunk and its motion, a backbone joint which can rotate in a horizontal axis was constructed. It imitates a connection between the first and second thoracic of a cockroach. Thus, it will provide enough mobility for the machine to climb over an obstacle to lift the front legs up to reach the top of an obstacle and then bend them downward during step climbing. All leg joints including the backbone joint are driven by digital servomotors [5].
The figure of cockroach' s climbing is modified from Ritzmann, R.E., Quinn,
R.D., and Fischer, M.S. 2004. Convergent evolution and locomotion through
complex terrain by insects, vertebrates and robots. Arthropod Structure and
Development, Vol. 33, No. 3, pp. 361–379.
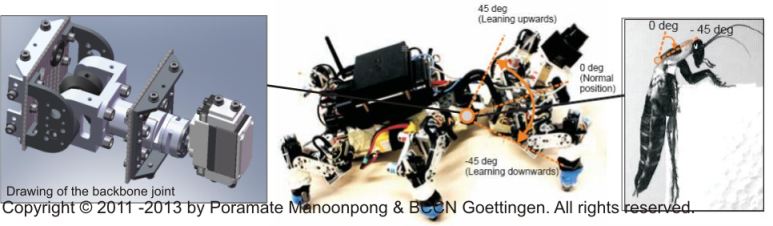
Muscles
The muscles are simulated based on a virtual agonist-antagonist mechanism
(VAAM) consisting of contractile and parallel elements (CEs and PEs). "Virtual"
here means that every joint physically actuated by a standard servo motor
can produce variably compliant motions as if it were driven by a pair of agonist
and antagonist muscles (see [3,8] for more detials).
Sensors
The AMOS II has a multitude of sensors: six foot contact sensors (FC), six reflexive optical sensors, seven infra-red sensors (IR), three light dependent resistor sensors (LDR), one upside-down detector sensor (UD), one inclinometer sensor (IM), one camera sensor (CM), one laser scanner sensor (LS), one current sensor (I). All these sensors are used to generate a broad behavioral repertoire including foothold searching, elevator reflex (swinging a leg over obstacles), self-protective reflex (standing in an upside-down position), obstacle avoidance, phototaxis (turn towards a light source), climbing over obstacles [1], five different gaits, and partially adaptation [5].
Different configurations based on the multi sensori-motor robotic platform
Due to our special design of a robot connector, one could reconfigure the joint angles of AMOS II or even simply reconstructure it to obtain different types, e.g., pentapod and octapod robots.
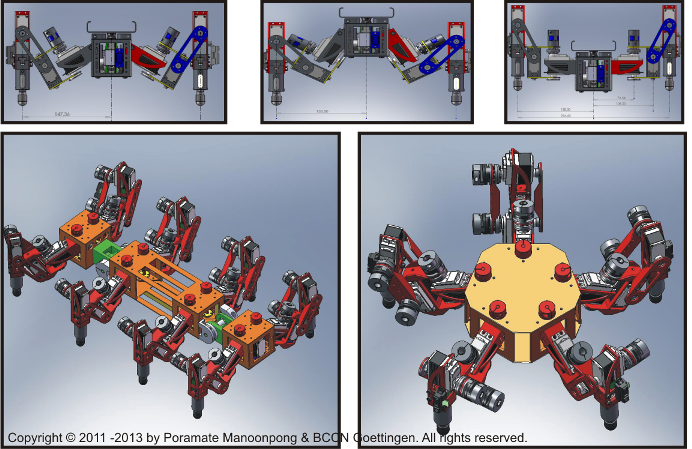
Adaptive neural locomotion control for insect-like, energy-efficient, and adaptable locomotion
The controller generates
insect-like, energy-efficient, and adaptable locomotion of AMOS II. This adaptive
neural closed-loop controller consists of one CPG-based control unit and
six local leg control units(read [5] for more details, Videos).
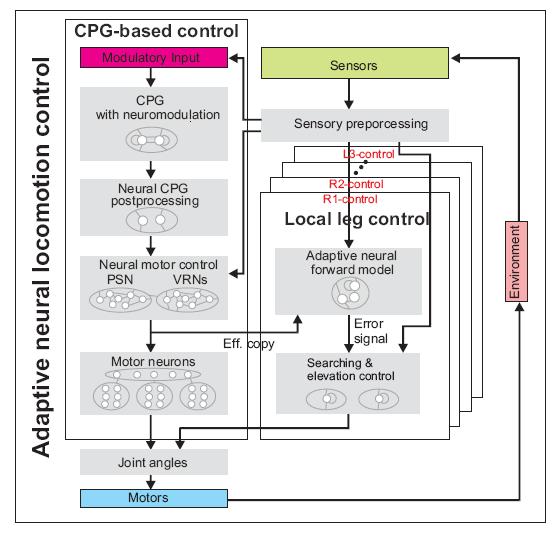
(For more details of the AMOS II and its controller, read the papers)
Self-adaptive reservoir (neural memory) for delay temporal memory tasks
We have developed self-adaptive reservoir. It is based on reservoir computing in which we have introduced intrinsic plasticity. It is aimed to be used as robot memory (see [4] for more details, Video).
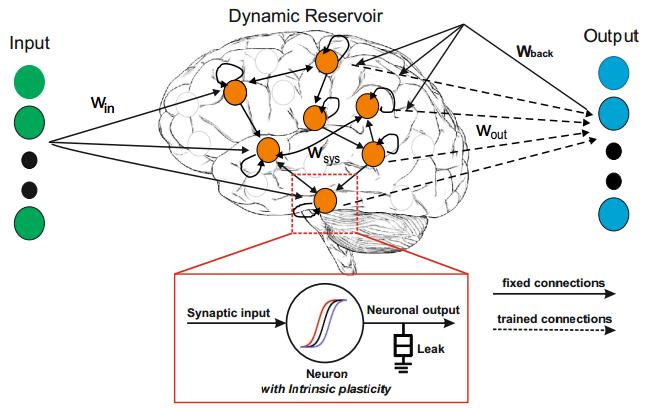
Virtual muscles for muscle-like behavior
We have developed a simplified variable admittance controller applied
to robot joint control. It is based on a virtual agonist-antagonist mechanism
(VAAM) consisting of contractile and parallel elements (CEs and PEs). "Virtual"
here means that every joint physically actuated by a standard servo motor
can produce variably compliant motions as if it were driven by a pair of agonist
and antagonist muscles.(see [3,8] for more details). Currently, the muscles' parameters for obtaining variable compliance are adjusted manually.
Reactive backbone joint control for climbing behavior
The reactive backbone joint control supports the climbing behavior of the robot by emulating the body flexion observed in cockroaches. The controller was developed and successfully tested using our modular robot control environment, allowing physical simulation and simple transferring to AMOS II. Experimental results show that employing this controller together with neural locomotion control and reflex mechanisms allows the robot to surmount obstacles with a maximum height of 13 cm which equals 75% of its leg length(see [1] for more details, Videos).
Multiple chaotic central pattern generators for locomotion generation
and leg damage compensation
Based on our previous development on chaotic CPG control, we have extended it to three CPGs, each of which controls a pair of opposite
legs of AMOS II. Our experimental results show that one CPG might not be enough to maintain the body balance and compensate for leg damage. Instead, using CPGs can cope with this problem (see [2] for more details, Video). Currently, finding proper parameters for leg damage compensation is achieved by manual adjustment in an offline manner; thereby fast and autonomous adaptation of an individual leg cannot be obtained in the current state.
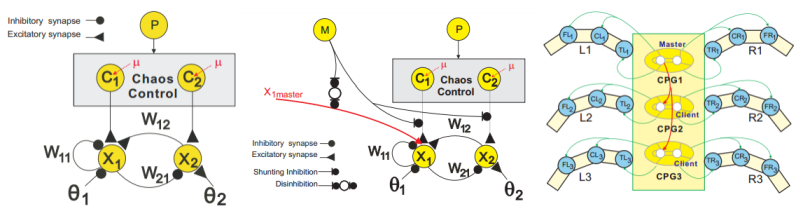
Performance of AMOS II
Energy efficient gait selections based on visual information (see [7] for more details, Video)
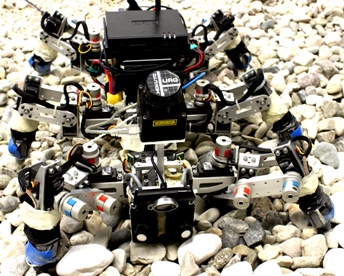
Obstacle/Gap Detection and Terrain Classification based on a 2D Laser Range Finder (see [9] for more details, Video)
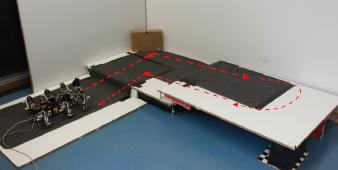
All Cmplex Locomotion Behaviors of AMOSII
(see [1-12] for more details,
Video)
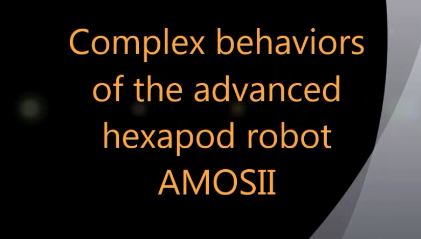
Description of AMOS II
Mechanics
Dimension without the tail (L x B x H): 40 x 30 x 20 cm
Weight: 5.8 Kg
Structure Carbon-fiber-reinforced polymer and Aluminum
alloys AL5083184
6 Legs with 3 degrees of freedom of each leg
Backbone joint rotating in a horizontal axis
All joints are driven by nineteen digital servomotors (2.9 Nm)
Electronics
Multi-Servo IO-Board (MBoard)1 developed at Fraunhofer Institute in Sankt Augustin. It is able to control up to 32 servomotors synchronously. At the same time 32 (+4 optional) analog input channels can be sampled and read with an update rate of up to 50 cycles per second.The board has an RS232 interface, which serves as the standard communication interface
Mini PC having an Intel Atom Z530 1.6GHz processor for programming neural mechanisms. It communicates with the MBoard via an RS232 interface
Batteries of 1x 11.1 V/3S-1P/3200 mAh (for servos), 2x 11.1
V/3S-1P/910 mAh (for MBoard/ sensors)
6 distance measurement infrared sensors (antenna-like sensors) located at the legs and the front part
Upside-down detector sensor, current sensor, etc
Programming
C programming on the MBoard for controlling servomotors and for reading digitized sensor data
C++ on the mini PC for programming neural preprocessing and control
Photos of AMOS II
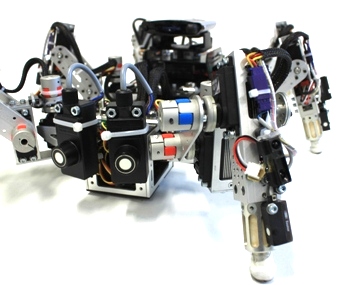 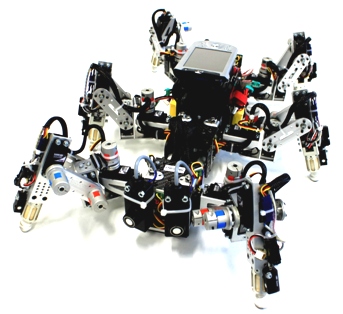
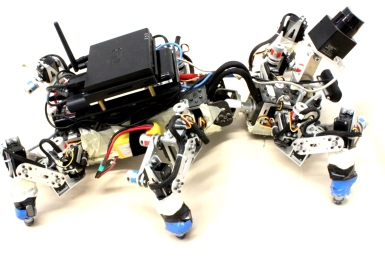 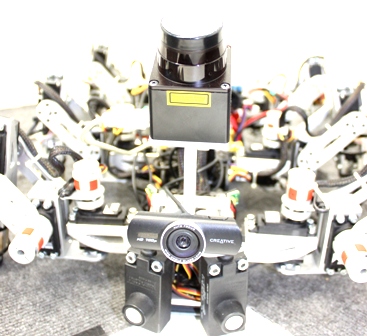
Copyright © 2011 -2013 by Poramate Manoonpong & BCCN Goettingen. All rights reserved.
Publications
[1]Goldschmidt, D.; Hesse, F. ; Wörgötter, F.; Manoonpong, P. (2012) Biologically Inspired Reactive Climbing Behavior of Hexapod Robots. IEEE/RSJ International Conference on Intelligent Robots and Systems (IROS) 2012, Protugal, pp. 4632-4637. (Best Paper Award Finalist and Best Student Paper Award Finalist). (pdf) [Supplmentary video]
[2]Ren, G.; Chen, W.; Kolodziejski, C.; Wörgötter, F.; Dasgupta, S.; Manoonpong, P. (2012) Multiple Chaotic Central Pattern Generators for Locomotion Generation and Leg Damage Compensation in a Hexapod Robot. IEEE/RSJ International Conference on Intelligent Robots and Systems (IROS) 2012, Protugal, pp. 2756-2761. (pdf) [Supplmentary video]
[3] Xiong, X.; Woergoetter, F. and Manoonpong, P.(2012) An Adaptive Neuromechanical Model for Muscle Impedance Modulations of Legged Robots. Dynamic Walking 2012 (DWC2012), May 21-25, 2012, Florida, United States .(pdf)
[4] Dasgupta, S.; Woergoetter, F.; Manoonpong, P. (2013) Information Dynamics based Self-Adaptive Reservoir for Delay Temporal Memory Tasks. Evolving Systems, DOI 10.1007/s12530-013-9080-y (pdf) (SJR = 1.51)
[5] Manoonpong, P.; Parlitz, U.; Wörgötter F. (2013) Neural Control and Adaptive Neural Forward Models for Insect-like, Energy-Efficient, and Adaptable Locomotion of Walking Machines. Front. Neural Circuits 7: 12. (pdf) DOI: 10.3389/fncir.2013.00012 (JIF = 5.098, SJR = 2.18) [Supplmentary video]
[6] Manoonpong, P.; Goldschmidt, D.; Woergoetter, F.;
Kovalev, A.; Heepe, L.; Gorb, S. (2013) Using a Biological Material to Improve Locomotion of Hexapod Robots. Living Machines: The International Conference on Biomimetics and Biohybrid Systems, London, UK, 29 July - 2 Aug 2013.
[7] Zenker, S.; Erdal Aksoy, E.; Goldschmidt, D.; Wörgötter, F.; Manoonpong, P. (2013) Visual Terrain Classification for Selecting Energy Efficient Gaits of a Hexapod Robot. The 2013 IEEE/ASME International Conference on Advanced Intelligent Mechatronics, Wollongong, Australia, 9-12 July 2013.
[8] Xiong, X.; Wörgötter, F.; Manoonpong, P. (2013) A Simplified Variable Admittance Controller Based on a Virtual Agonist-Antagonist Mechanism for Robot Joint Control. 16th International Conference on Climbing and Walking Robots and the Support Technologies for Mobile Machines (CLAWAR), University of Technology, Sydney, Australia, 14 – 17 July 2013.
[9] Kesper, P.; Grinke, E.; Hesse, F.; Wörgötter, F.; Manoonpong, P. (2013) Obstacle/Gap Detection and Terrain Classification of Walking Robots based on a 2D Laser Range Finder. 16th International Conference on Climbing and Walking Robots and the Support Technologies for Mobile Machines (CLAWAR), University of Technology, Sydney, Australia, 14 – 17 July 2013.
[10] Xiong, X.; Woergoetter, F.;
Manoonpong, P. (2015) Adaptive and Energy Efficient
Walking in a Hexapod Robot under Neuromechanical Control
and Sensorimotor Learning, IEEE Transactions on
Cybernetics, 99, DOI: 10.1109/TCYB.2015.2479237 (JIF =
3.469 , SJR = 1.56) [Supplemetary video]
[11] Grinke, E.; Tetzlaff, C.;
Wörgötter, F.; Manoonpong, P. (2015) Synaptic
plasticity in a recurrent neural network for versatile and
adaptive behaviors of a walking robot. Front.
Neurorobot. 9:11. doi: 10.3389/fnbot.2015.00011
[Supplementary video] (SJR = 0.66)
[12] Dasgupta,
S.; Goldschmidt, D.; Woergoetter, F.; Manoonpong, P.
(2015) Distributed Recurrent Neural Forward Models with
Neural Control for Complex Locomotion in Walking Robots.
Front. Neurorobot. 9:10.DOI: 10.3389/fnbot.2015.00010 (SJR
= 0.66)
[13] Ren, G.; Chen, W.; Dasgupta,
S.; Kolodziejski, C.; Wörgötter, F.; Manoonpong, P. (2015)
Multiple Chaotic Central Pattern Generators with
Learning for Legged Locomotion and Malfunction
Compensation. Information Sciences 294, 666-682
[14] Manoonpong, P.; Petersen, D.;
Kovalev, A.; Woergoetter, F.; Gorb, S.; Spinner, M.;
Heepe, L. (2016) Enhanced Locomotion Efficiency of a
Bio-inspired Walking Robot using Contact Surfaces with
Frictional Anisotropy, Scientific Reports 6(39455)
doi:10.1038/srep39455, Nature Publishing
|
News of AMOS II
2017
2013
Top of Page
|
|